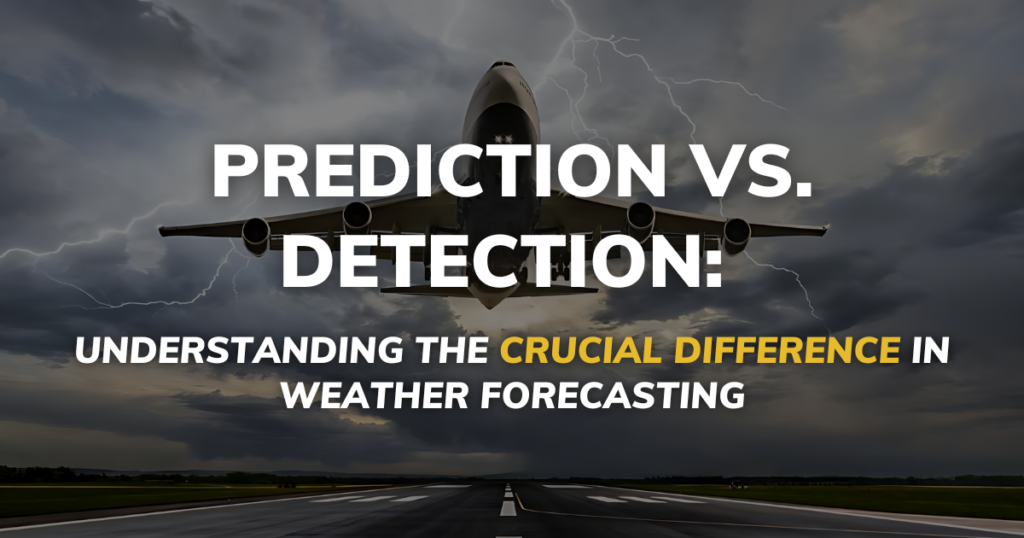
Prediction vs. Detection: Understanding the Crucial Difference in Weather Forecasting
Prediction vs. Detection: A Critical Contrast in Modern Science of the Weather
Let’s explore the crucial differences between prediction and detection in modern weather science and how Flash Weather AI leverages machine learning to improve forecasting accuracy.
Imagine this. It’s a warm spring day. When you walk outside and look up at the blue sky with its bunches of fluffy clouds, the forecast is for rain, but it does not seem to make all that much difference because the sun feels so lovely. This contrast highlights the importance of accurate weather prediction and detection, especially in the context of lightning prediction. In this blog, we delve into the crucial differences between prediction and detection in modern weather science and how Flash Weather AI leverages machine learning to better the accuracy of forecasting.
Traditional Weather Prediction Limits: Limitations of Traditional Weather Forecasting
Traditional weather predictions take on a glow-through analysis of current weather conditions and atmospheric models to specify changes. However, they have their limitations:
- Overlooking Rapid Developments: Traditional models often miss rapidly developing or localized weather conditions crucial for accurate lightning prediction and detection.
- Historical Data Limitations: They lack the ability to incorporate particular data from the environment. And do not incorporate years of post-data for continued analysis.
- Accuracy Decline Over Time: Forecast accuracy significantly drops beyond 3 days due to increasing uncertainty.
For example, traditional forecasts would say the day will be bright and sunny, but then an afternoon thunderstorm with heavy rainfall and intense lightning may occur.
How Machine Learning Improves Weather Forecasting: Enhancing Accuracy with AI
Machine learning transforms weather forecasting by:
- Detecting Complex Patterns: This technique analyzes vast years of datasets to find subtle links not apparent to humans, which is particularly useful for lightning prediction.
- Adapting to Changing Conditions: Machine learning models continuously learn from new data, providing updated and more accurate predictions
- Accounting for Uncertainty: AI performs multiple simulations to find the similarities of past weather events applying the best outcome all compiled in nanoseconds, enhancing the accuracy of forecasts.
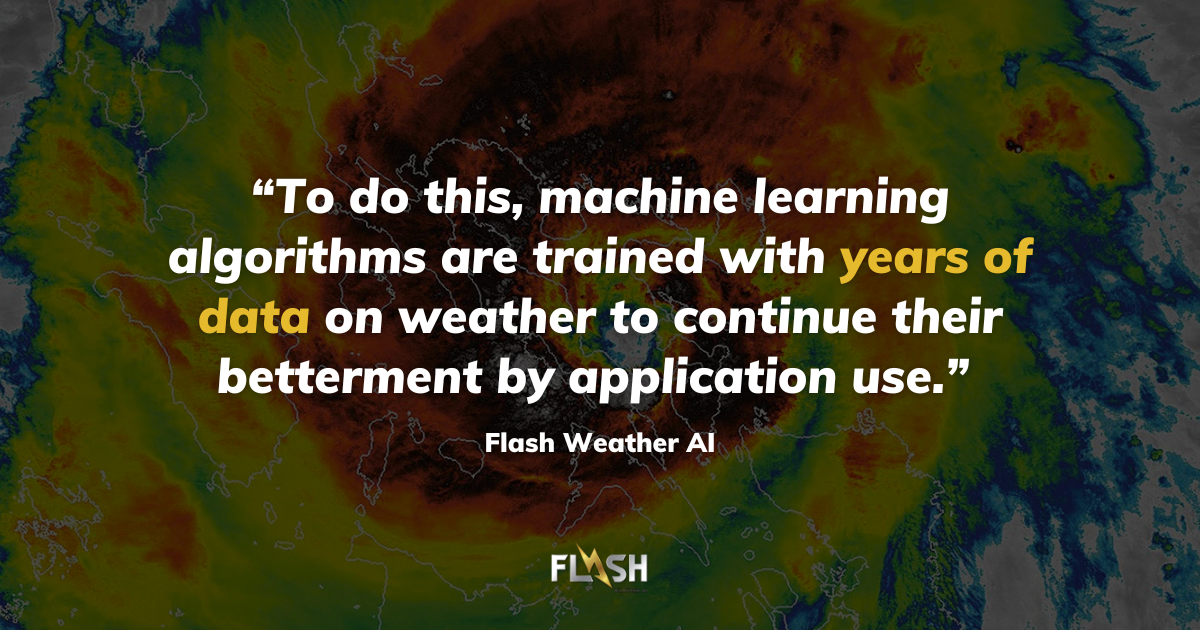
Integrating Prediction and Detection through AI For Flash Weather: Improved Forecasting
Weather forecasting has evolved from a probability and prediction model to the use of sophisticated instruments to receive real-time data. By incorporating both concepts—the predictive simulation and current atmospheric observations—meteorologists are better placed to get broader and more precise ideas on the weather.
Predictive model integration with real-time detection works thus:
- Numerical Weather Prediction Models: The assimilation process uses high computational power, allowing the product for up to 100 km. , and for multiple days out.
- Weather Detection Systems: Using instruments like weather satellites and Doppler radar offers real-time information and, hence, more accurate forecasts.
- Flash Weather Prediction: Takes more data in, than those standard numerical weather prediction models to provide an all-encompassing weather prediction. We start by collecting hundreds and hundreds of data points every millisecond to every hour from the following data sources:
-
- Radar (NEXRAD)
- Model data
- Satellite
- Observations
We do not wait, like some models, wait 1 - 6 hours, we process and provide new predictive data every 2 minutes via our proprietary patented algorithm, then we apply AI, including machine learning and deep learning, to give you the most accurate prediction you will experience.
The AI marks a “turning point” for weather forecasting and decision-making, the scientists at Google DeepMind say.
The key is more recognition of the difference between prediction and detection, better integration of modern meteorology, and using both techniques. These methods will then show how important it is to achieve the correct accuracy and alertness, which are modern methods of weather forecasting.
Using AI and machine learning is the best way to achieve more accuracy. Such integrated forecasting with real-time detection changes weather science as much as AI. It's not only an application: It is a system based on both guiding predictive algorithms and a sense of broader meteorological studies.
Combining these elements offers the public the most accurate and timely weather forecasts, driving advancement and continued innovation in this critical field of science.
By understanding the difference between prediction and detection, particularly in the context of lightning prediction, we can better prepare for whatever the sky holds. Flash Weather AI's advanced weather intelligence keeps you ahead of the weather, integrating predictive algorithms and real-time detection methods.
Visit FlashWeather.ai to learn how our advanced weather intelligence can keep you ahead of the weather.
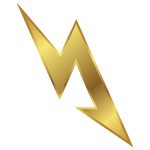
Jamie Castle
Flash Weather AI: VP of Sales and COO.
Jamie, the VP of Sales and COO of Flash Weather AI, brings 26 years of meteorology experience from NetJets Inc., where they served in roles including Chief Meteorologist and Flight Operations Mitigation Expert. He is an honorably discharged veteran of the United States Air Force.
Get a Live Demo today to see Flash Weather AI in action!